Basic Information
Position: | Chancellor's Associate Professor, School of Information and Public Policy Director, Global Policy Lab Faculty Co-Director, Center for Effective Global Action University of California, Berkeley | ![]() |
Contact: | jblumenstock[@]berkeley[.]edu | |
My work focuses on using novel data and methods to try and improve the lives of disadvantaged people around the world. You can find a short professional bio here. |
Featured Papers
Björkegren, D, Blumenstock, JE, and Knight, S (2025). Learning what Policies Value, Review of Economic Studies (Accepted)
When a policy prioritizes one person over another, is it because they benefit more, or because they are preferred? This paper develops a method to uncover the values consistent with observed allocation decisions. We estimate how much each individual benefits from an intervention, and then reconcile the allocation with (i) the welfare weights assigned to different people; (ii) heterogeneous treatment effects of the intervention; and (iii) weights on different outcomes. Analyzing Mexico's PROGRESA anti-poverty program, we find that while the program prioritized certain subgroups - such as indigenous households - the fact that those groups benefited more implies that the program did not actually assign them a higher welfare weight. The PROGRESA case illustrates how the method makes it possible to audit existing policies, and to design future policies that better align with values.
Blumenstock, JE, Chi, G, and Tan, X (2025). Migration and the Value of Social Networks, Review of Economic Studies, 92(1):97-128 [pdf]
How do social networks influence the decision to migrate? Prior work suggests two distinct mechanisms that have historically been difficult to differentiate: as a conduit of information, and as a source of social and economic support. We disentangle these mechanisms using a massive 'digital trace' dataset that allows us to observe the migration decisions made by millions of individuals over several years, as well as the complete social network of each person in the months before and after migration. These data allow us to establish a new set of stylized facts about the relationship between social networks and migration. Our main analysis indicates that the average migrant derives more social capital from 'interconnected' networks that provide social support than from 'extensive' networks that efficiently transmit information.
Aiken, E, Bellue, S, Karlan, D, Udry, C, and Blumenstock, JE (2022). Machine Learning and Phone Data Can Improve the Targeting of Humanitarian Aid, Nature, 603: 864-870 [pdf]
Targeting is a central challenge in the administration of anti-poverty programs: given available data, how does one rapidly identify the individuals and families with the greatest need? Here we show that non-traditional “big” data from satellites and mobile phone networks can improve the targeting of anti-poverty programs. Our analysis compares outcomes – including exclusion errors, total social welfare, and measures of fairness – under different targeting regimes. Relative to other feasible targeting options, the machine learning approach reduces errors of exclusion by 4-21%. These results highlight the potential for new data sources to contribute to humanitarian response efforts, particularly in crisis settings when traditional data are missing or out of date.
Chi, G, Fang, S, and Blumenstock, JE (2022). Microestimates of Wealth and Poverty for all Low- and Middle-Income Countries, Proceedings of the National Academy of Sciences, 119(3), 1-11 [pdf]
Many critical policy decisions rely on data about the geographic distribution of wealth and poverty. Yet many poverty maps are out of date or exist only at very coarse levels of granularity. Here we develop the first microestimates of wealth and poverty that cover the populated surface of all 135 low and middle-income countries (LMICs) at 2.4km resolution. The estimates are built by applying machine learning algorithms to vast and heterogeneous data from satellites, mobile phone networks, topographic maps, as well as aggregated and de-identified connectivity data from Facebook. We train and calibrate the estimates using nationally-representative household survey data from 56 LMICs, then validate their accuracy using four independent sources of household survey data from 18 countries. We also provide confidence intervals for each microestimate to facilitate responsible downstream use...
Rolf, Simchowitz, Dean, Liu, Björkegren, Hardt, and Blumenstock (2020). Score-Based Classifiers for Welfare-Aware Machine Learning, International Conference on Machine Learning (ICML '20) [pdf]
While real-world decisions involve many competing objectives, algorithmic decisions are often evaluated with a single objective function. We study algorithmic policies which explicitly trade off between a private objective (such as profit) and a public objective (such as social welfare). We analyze a natural class of policies which trace an empirical Pareto frontier based on learned scores, and focus on how such decisions can be made in noisy or data-limited regimes. Our theoretical results characterize the optimal strategies in this class, bound the Pareto errors due to inaccuracies in the scores, and show an equivalence between optimal strategies and a rich class of fairness-constrained profit-maximizing policies.
View all publications...
Featured Ongoing Projects
Manipulation-Robust Prediction - joint with Daniel Björkegren and Samsun Knight (Revise and Resubmit, American Economic Review)
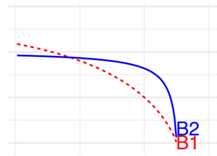
An increasing number of decisions are guided by machine learning algorithms. But when consequential decisions are encoded in algorithms, individuals may strategically alter their behavior to achieve desired outcomes. This paper develops an empirical approach to adjust decision algorithms to anticipate manipulation. By explicitly modeling incentives to manipulate, our approach produces decision rules that are stable under manipulation, even when the rules are fully transparent. We stress test this approach through a large field experiment in Kenya. When implemented, decision rules estimated with this strategy-robust approach outperform those based on standard machine learning approaches.
Strengthening Fragile States: Evidence from Mobile Salary Payments in Afghanistan - joint with Michael Callen, Anastasia Faikina, Stefano Fiorin, Tarek Ghani (Revise and Resubmit, Review of Economic Studies)
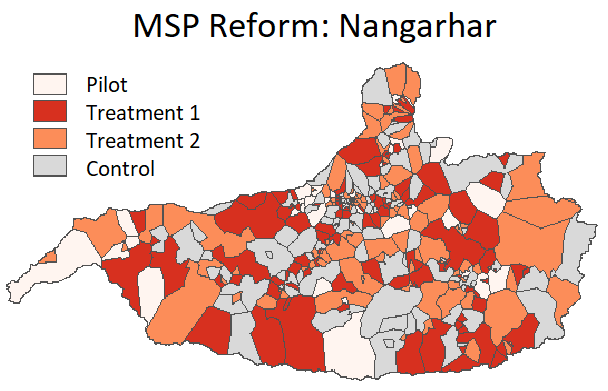
We conduct a randomized evaluation of a flagship Afghan government initiative aimed at building state administrative capacity during a period when the state faced existential uncertainty. The program aimed to modernize employee tracking and salary payments in the Ministry of Education through biometric registration and digital payment systems. The reform reduced payment delays, decreased teacher turnover, modestly improved student learning outcomes, and expanded financial inclusion. Employee enrollment was higher in districts where citizens had greater confidence in and consensus about the government’s prospects to defeat the Taliban.
Measuring Religion from Behavior: Climate Shocks and Religious Adherence in Afghanistan - joint with Oeindrila Dube and Michael Callen
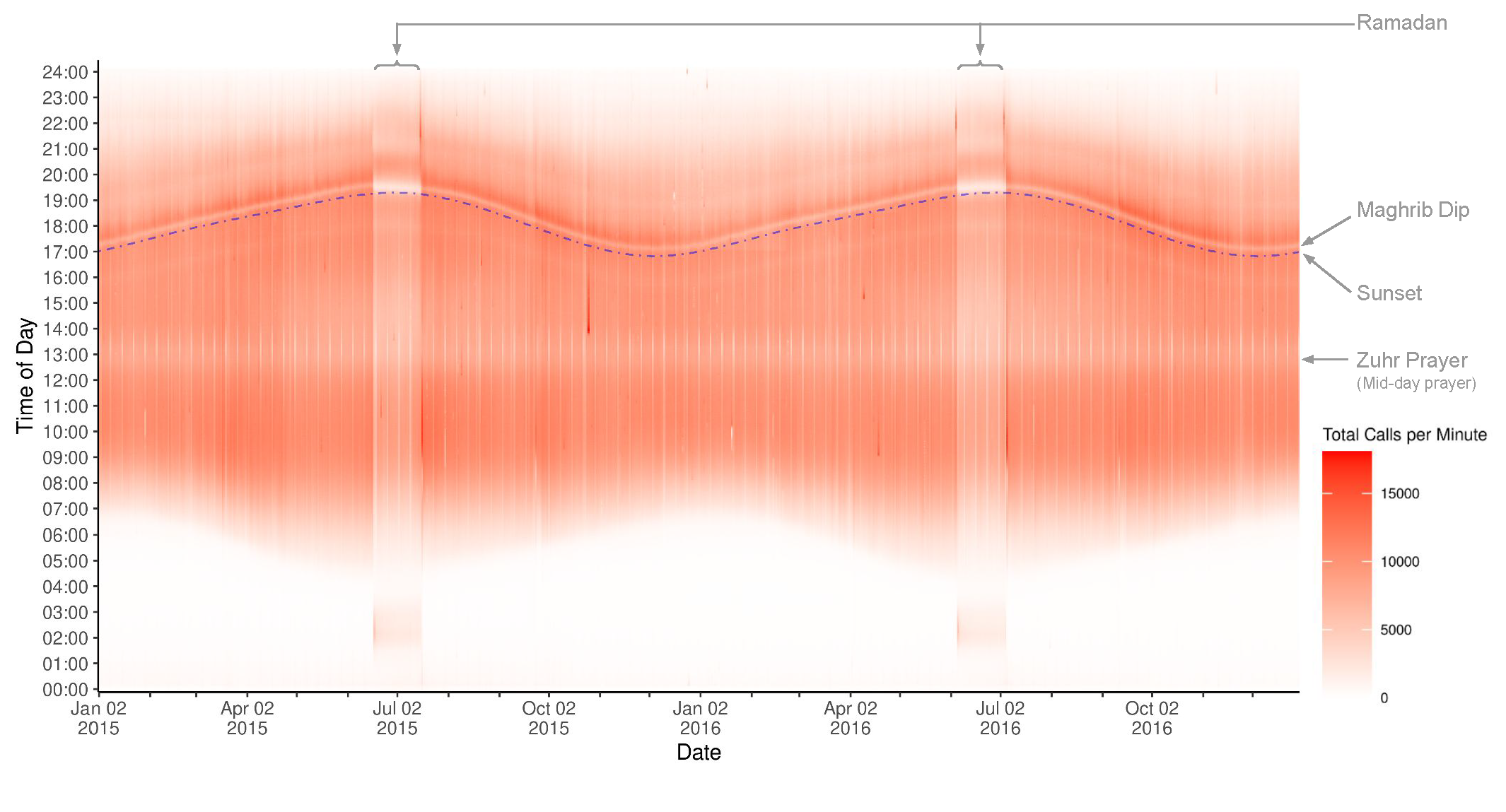
Religious adherence has been hard to study in part because it is hard to measure. We develop a new measure of religious adherence, which is granular in both time and space, using anonymized mobile phone transaction records. After validating the measure with traditional data, we show how it can shed light on the nature of religious adherence in Islamic societies. Exploiting random variation in climate, we find that as economic conditions in Afghanistan worsen, people become more religiously observant. The effects are most pronounced in areas where droughts have the biggest economic consequences, such as croplands without access to irrigation.
Insecurity and Firm Displacement: Evidence from Afghan Corporate Phone Records - joint with Tarek Ghani, Sylvan Herskowitz, Ethan B. Kapstein, Thomas Scherer, and Ott Toomet (Conditionally Accepted, American Economic Journal: Economic Policy)
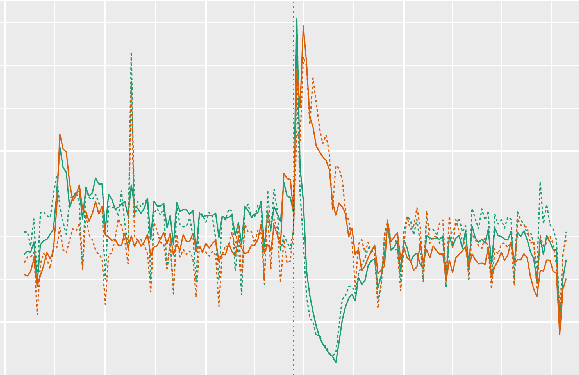
We provide new evidence on how insecurity affects firm behavior by linking data on violent conflict in Afghanistan to geo-stamped corporate mobile phone records. We begin by developing a method for observing firm location choice with phone data, and validate these measurements using independent sources of administrative and survey data. Next, we show that deadly terrorist attacks reduce the presence of firms in targeted districts by 4-6%. The effect includes both an increase in the local exit of existing firms following attacks and a decrease in new firm entry. We find large negative spillovers from attacks in provincial capitals on firm presence in nearby rural districts. After violence, employees in provincial capitals are 33% more likely to move to Kabul and 15% more likely to leave for another province.
Yellow Pages: Information, Connections, and Firm Performance - joint with Brian Dillon and Jenny Aker (Accepted, Economic Journal)
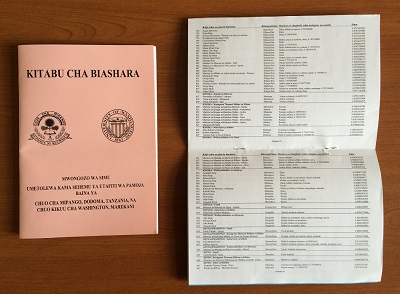
Mobile phones reduce the cost of communicating with existing social contacts, but do not eliminate frictions in forming new relationships. We report the findings of a two-sided randomized control trial in central Tanzania, centered on the production and distribution of a "yellow pages" phone directory with contact information for local enterprises. Enterprises randomly assigned to be listed in the directory receive more business calls, make more use of mobile money, and employ more workers. There is evidence of positive spillovers, as both listed and unlisted enterprises in treatment villages experience significant increases in sales relative to a pure control group. Households randomly assigned to receive copies of the directory make greater use their phones for farming, are more likely to rent land and hire labor, have lower rates of crop failure, and sell crops for weakly higher prices. Willingness-to-pay to be listed in future directories is significantly higher for treated enterprises.
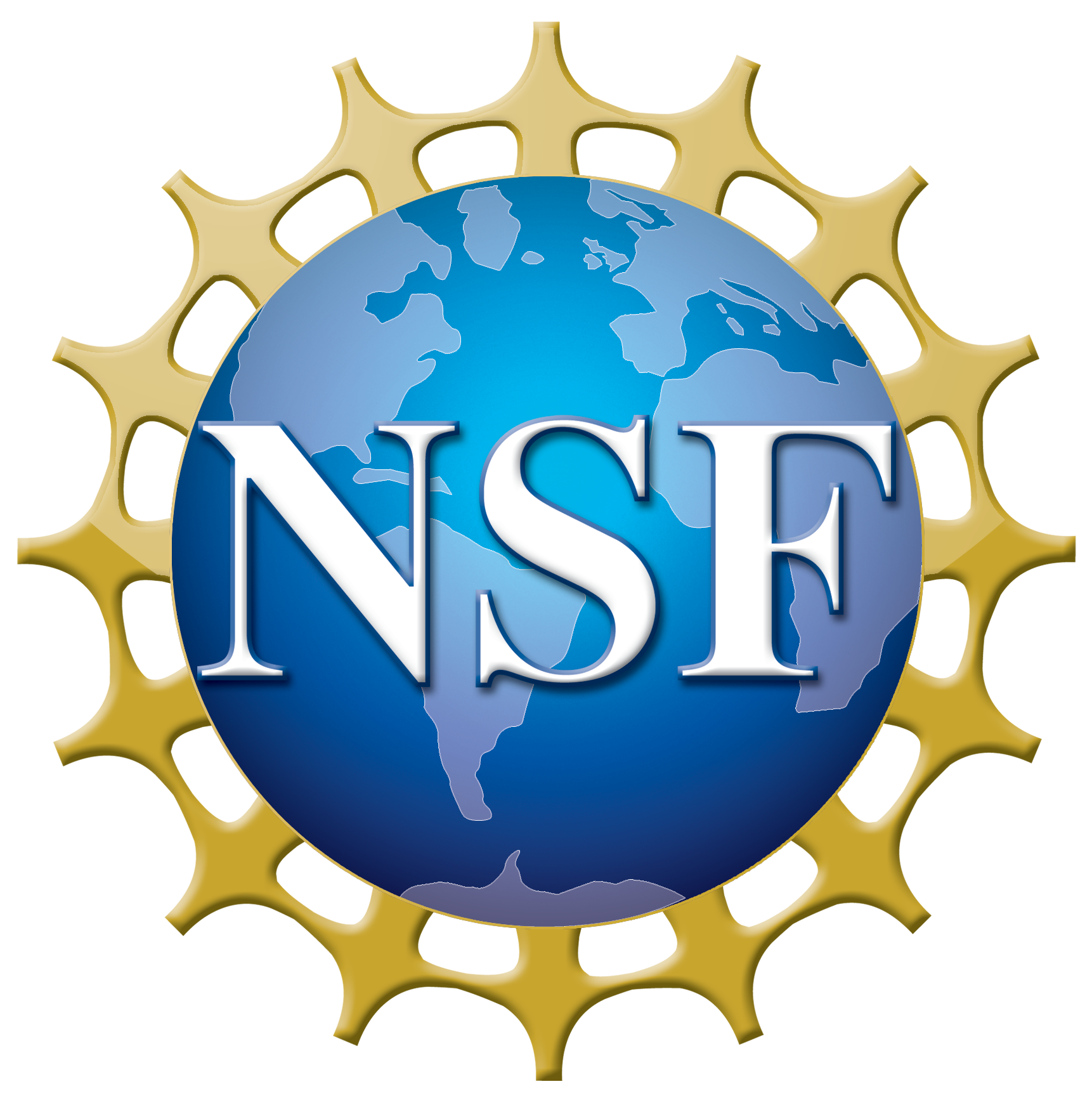
Many decisions that once were made by humans are now made using algorithms. These algorithms are typically designed with a single, profit-related objective in mind: Loan approval algorithms are designed to maximize profit, smart phone apps are optimized for engagement, and news feeds are optimized for clicks. However, these decisions have side effects: irresponsible payday loans, addictive apps, and fake news can harm individuals and society. This project develops and tests a new paradigm for prioritizing the social impact of an algorithmic decision from the start, rather than as an afterthought. The key insight is to leverage recent advances in machine learning -- which make it possible to predict who will benefit from a decision and how -- to design algorithms that balance those predicted benefits alongside traditional profit-related objectives.
Instant Loans Can Lift Subjective Well-Being: A Randomized Evaluation of Digital Credit in Nigeria - joint with Daniel Björkegren, Omowunmi Folajimi-Senjobi, Jacqueline Mauro, and Suraj Nair (Accepted, Economic Development and Cultural Change)
Digital loans have exploded in popularity across low- and middle-income countries, providing short term, high interest credit via mobile phones. This paper reports the results of a randomized evaluation of a digital loan product in Nigeria. Being randomly approved for a loan (among those who otherwise would have been denied) substantially increases subjective well-being after an average of three months, but being randomly approved for a larger loan does not have any eect. Neither intervention signicantly impacts other measures of welfare, and we can rule out large impacts - either positive or negative - on income and expenditures, resilience, and women's economic empowerment.
The Impact of Mobile Phones: Experimental Evidence from the Random Assignment of New Cell Towers - joint with Niall Keleher, Arman Rezaee, Erin Troland
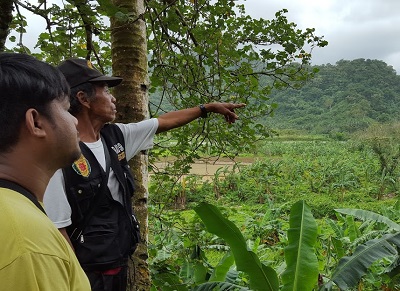
We present experimental evidence on the economic impacts of mobile phone access. Our results are based on a randomized control trial in the Philippines, through which 14 isolated and previously unconnected villages were randomly assigned to either receive or not receive a new cellphone tower. Following a pre-analysis plan, we find that the introduction of mobile phones had large and significant impacts on household income and expenditure, particularly for wage workers. Mobile phone access also increased social connections within and between communities. However, there are no consistent impacts on market access, informedness, or subjective well being. In post-specified analysis, we find suggestive evidence that the improved economic conditions are driven by increases in migration, remittances, and self-employment. Working paper available by request.